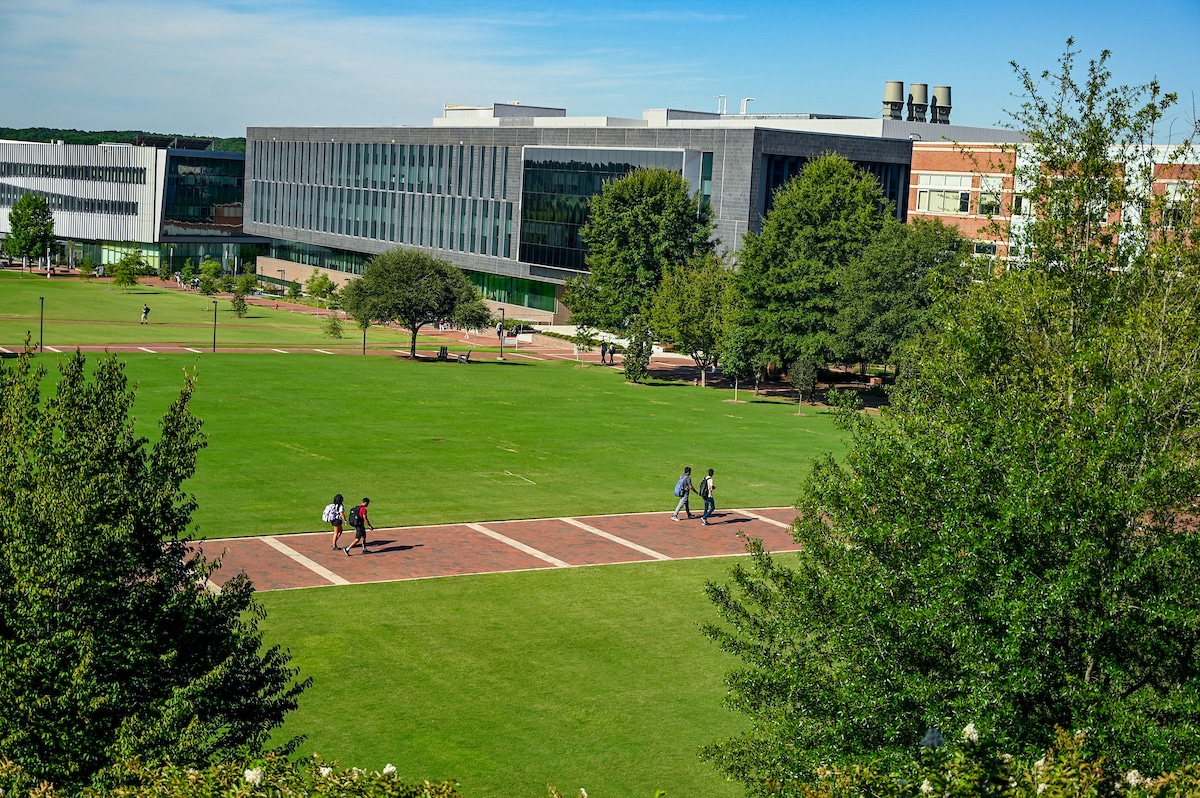
Electrical and computer engineering Ph.D. students, Priyank Kashyup and Yuejiang Wen, each won the Best Poster Award at CAEML’s Fall 2022 Semiannual Meeting. The posters and presentations are voted on by the Industry members in attendance at the meeting and presented by CAEML Director Elyse Rosenbaum at the Semiannual Meeting Dinner.
The mission of CAEML is to enable fast, accurate design and verification of microelectronic circuits and systems by creating machine learning algorithms to derive models used for electronic design automation (EDA), resulting in a reduced design cycle time and radically improved design reliability.
Priyank Kashyup’s project aims to use generative networks to develop an entirely data-driven approach for complex models for mixed-signal circuits. They use a unique transformation that represents the time-series data as an image which keeps the temporal dependencies between different time steps, a vital feature of these models.
With this transformation, the model can predict the eye diagram or bit error contour plot, a measure of whether a receiver can sample data while handling different input patterns over channel conditions and account for unseen device configurations. Through this process, they can generate models that correlate to actual device measurements while taking a fraction of the development time.
Such a machine learning model scheme would enable intellectual property (IP) vendors to share information about their devices quicker and have them represent the real-world device. This project builds on the prior work on time series to image transformations for hardware side-channel attacks under Aydin Aysu, ECE assistant professor. It leverages the expertise of NC State machine learning ECE faculty, Tianfu Wu, Chau-Wai Wong, and Dror Baron, as well as our industry mentors, Chris Cheng and Yongjin Choi, from Hewlett Packard Enterprise.
Yuejiang Wen, mentored by Paul Franzon, won this award for his work on ‘High Dimensional Optimization for Electronic Design.’ Wen’s research focus is to develop automatic high-dimensional optimization tools that can optimize electronic designs efficiently and effectively. The Inspection-based random embedding Bayesian Optimization (IC-REMBO) was proposed to tackle this challenge and demonstrated effectiveness in optimizing high-dimension analog circuits. This project collaborates with co-PIs and ECE faculty members, Dror Baron and Brian Floyd.