Researchers have developed an artificial intelligence (AI) program that can automatically provide species-level identification of microscopic marine organisms. The next step is to incorporate the AI into a robotic system that will help advance our understanding of the world’s oceans, both now and in our prehistoric past.
Specifically, the AI program has proven capable of identifying six species of foraminifera, or forams – organisms that have been prevalent in Earth’s oceans for more than 100 million years.
Forams are protists, neither plant nor animal. When they die, they leave behind their tiny shells, most less than a millimeter wide. These shells give scientists insights into the characteristics of the oceans as they existed when the forams were alive. For example, different types of foram species thrive in different kinds of ocean environments, and chemical measurements can tell scientists about everything from the ocean’s chemistry to its temperature when the shell was being formed.
However, evaluating those foram shells and fossils is both tedious and time consuming. That’s why an interdisciplinary team of researchers, with expertise ranging from robotics to paleoceanography, is working to automate the process.
“At this point, the AI correctly identifies the forams about 80 percent of the time, which is better than most trained humans,” says Edgar Lobaton, an associate professor of electrical and computer engineering at North Carolina State University and co-author of a paper on the work.
“But this is only the proof of concept. We expect the system to improve over time, because machine learning means the program will get more accurate and more consistent with every iteration. We also plan to expand the AI’s purview, so that it can identify at least 35 species of forams, rather than the current six.”
The current system works by placing a foram under a microscope capable of taking photographs. An LED ring shines light onto the foram from 16 directions – one at a time – while taking an image of the foram with each change in light. These 16 images are combined to provide as much geometric information as possible about the foram’s shape. The AI then uses this information to identify the foram’s species.
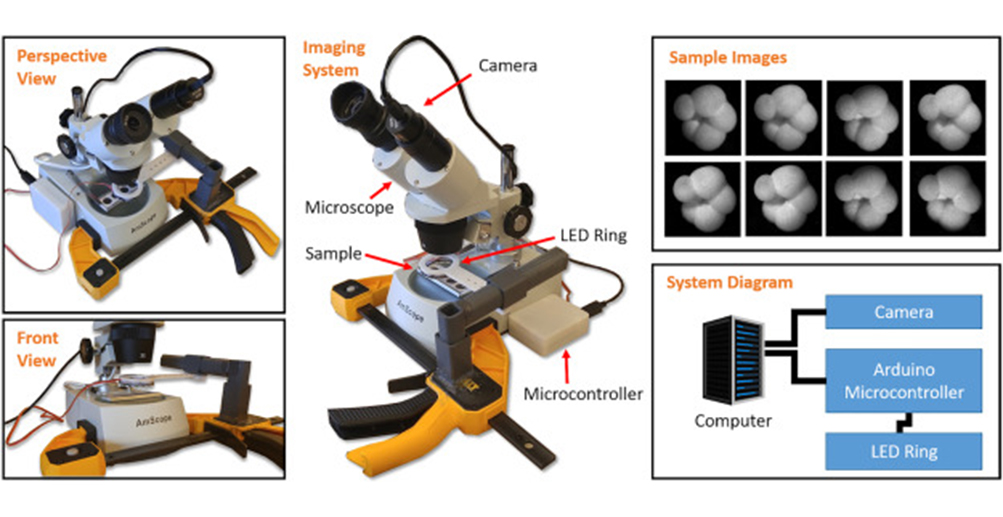
The scanning and identification takes only seconds, and is already as fast – or faster – than the fastest human experts.
“Plus, the AI doesn’t get tired or bored,” Lobaton says. “This work demonstrates the successful first step toward building a robotic platform that will be able to identify, pick and sort forams automatically.”
Lobaton and his collaborators have received a grant from the National Science Foundation (NSF), starting in January 2019, to build the fully-functional robotic system.
“This work is important because oceans cover about 70 percent of Earth’s surface and play an enormous role in its climate,” says Tom Marchitto, an associate professor of geological sciences at the University of Colorado, Boulder, and corresponding author of the paper.
“Forams are ubiquitous in our oceans, and the chemistry of their shells records the physical and chemical characteristics of the waters that they grew in. These tiny organisms bear witness to past properties like temperature, salinity, acidity and nutrient concentrations. In turn we can use those properties to reconstruct ocean circulation and heat transport during past climate events.
“This matters because humanity is in the midst of an unintentional, global-scale climate ‘experiment’ due to our emission of greenhouse gases,” Marchitto says. “To predict the outcomes of that experiment we need a better understanding of how Earth’s climate behaves when its energy balance is altered. The new AI, and the robotic system it will enable, could significantly expedite our ability to learn more about the relationship between the climate and the oceans across vast time scales.”
The paper, “Automated species-level identification of planktic foraminifera using convolutional neural networks, with comparison to human performance,” is published in the journal Marine Micropaleontology. Lead author of the paper is Ritayan Mitra, a former postdoctoral researcher at NC State and the University of Colorado Boulder, who is now at IIT Bombay. Co-authors include Q. Ge and B. Zhong, Ph.D. students at NC State; B. Kanakiya, a former master’s student at NC State; M.S. Cook of Williams College; J.S. Fehrenbacher of Oregon State University; J.D. Ortiz of Kent State University; and A. Tripati of UCLA.
The AI work was done with support from NSF under grant number 1637039.
-shipman-
Note to Editors: The study abstract follows.
“Automated species-level identification of planktic foraminifera using convolutional neural networks, with comparison to human performance”
Authors: R. Mitra and T.M. Marchitto, University of Colorado, Boulder; Q. Ge, B. Zhong, B. Kanakiya and E. Lobaton, North Carolina State University; M.S. Cook, Williams College; J.S. Fehrenbacher, Oregon State University; J.D. Ortiz, Kent State University; and A. Tripati, Indian Institute of Technology
Published: Jan. 25, Marine Micropaleontology
DOI: 10.1016/j.marmicro.2019.01.005
Abstract: Picking foraminifera from sediment samples is an essential, but repetitive and low-reward task that is well-suited for automation. The first step toward building a picking robot is the development of an automated identification system. We use machine learning techniques to train convolutional neural networks to identify six species of extant planktic foraminifera that are widely used by paleoceanographers. Identification includes distinguishing the six species from other taxa. Training and identification are based on reflected light microscope digital images taken at 16 different illumination angles using a light ring. Overall machine accuracy is better than 80% even with limited training. We compare machine performance to that of human pickers (six experts and five novices) by tasking each with the identification of 540 specimens based on images. Experts achieved comparable precision but poorer recall relative to the machine, with an average accuracy of 63%. Novices scored lower than experts on both precision and recall, for an overall accuracy of 53%. The machine achieved fairly uniform performance across the six species, while participants’ scores were strongly species-dependent, commensurate with their past experience and expertise. The machine was also less sensitive to specimen orientation (umbilical versus spiral views) than the humans. These results demonstrate that our approach can provide a versatile ‘brain’ for an eventual automated robotic picking system.
This post was originally published in NC State News.